
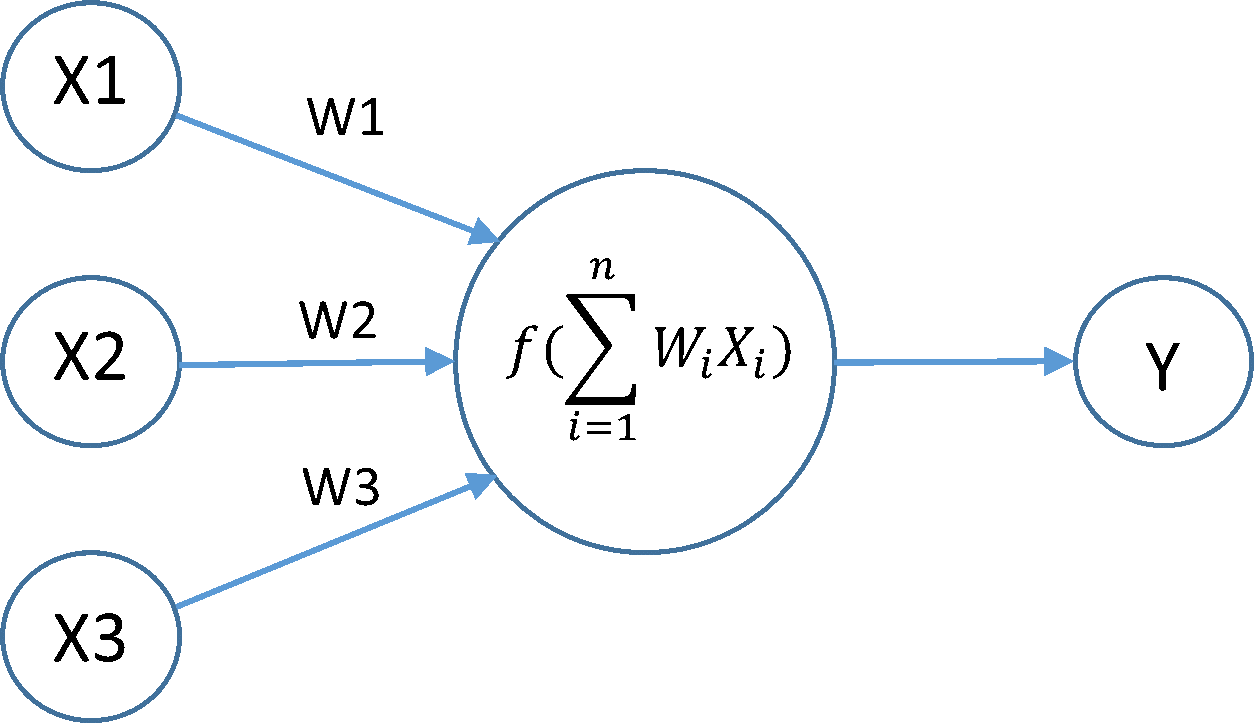
ANN can be classified into feedforward and feedback network. ANN is widely used in many areas because of its features such as strong capacity of nonlinear mapping, high accuracy for learning, and good robustness. It is an adaptive system that changes its structure or internal information that flows through the network during the training phase. The issue arises because the network matches the data so closely as to lose its generalization ability over the test data.Īrtificial neural networks (ANN) is an information processing system which is inspired by the models of biological neural networks. Over training is akin to the issue of overfitting data. There exists an overtraining issue in the design of NN training process. This is very important while the neural network is trained to get very small errors which may not respond properly in wind speed prediction.

One of the major problems facing researchers is the selection of hidden neurons using neural networks (NN). The proposed model is simple, with minimal error, and efficient for fixation of hidden neurons in Elman networks. The survey has been made for the fixation of hidden neurons in neural networks. The experimental results show that with minimum errors the proposed approach can be used for wind speed prediction. To verify the effectiveness of the model, simulations were conducted on real-time wind data. The perfect design of the neural network based on the selection criteria is substantiated using convergence theorem. The results show that proposed model improves the accuracy and minimal error. To fix hidden neurons, 101 various criteria are tested based on the statistical errors. This paper proposes the solution of these problems. The random selection of a number of hidden neurons might cause either overfitting or underfitting problems. And it also proposes a new method to fix the hidden neurons in Elman networks for wind speed prediction in renewable energy systems. This paper reviews methods to fix a number of hidden neurons in neural networks for the past 20 years.
